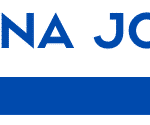
International Labour Organization
Contract’s duration: 20 October 2024to 20 December 2024
Total Working Days: 35 Days
Background
The success of policies aimed at Universal Social Protection depends critically on the use of Information and Communications Technology (ICT) and data, and their effective governance and management. Data quality is critical to realizing the potential of digital transformation.
A well-functioning management information system depends on its data quality foundations. Without proper data quality, businesses risk making decisions based on flawed or incomplete information, leading to wasted resources, ineffective strategies, and dissatisfied customers Data quality management supposes, concurrently with digital transformation, specifying information and data needs, data dictionaries, data quality requirements, profiling, analyzing, and assessing the quality of existing data, correcting data quality defects, and preparing for data migration. It also encompasses defining procedures for managing data quality issues once new information systems including management information systems are in place. Ultimately, the good administration of management information systems requires proper data governance systems and processes. Data governance frameworks should follow international standards and practices and technical specifications (e.g. ISO/IEC 38500, COBIT® 4.1, COBIT® 5 and international practices defined in ISO 8000 and the DAMA-DMBOK2). This entails setting up inter alia a data quality framework, a stewardship program, and organizational structures to carry out data governance processes.
Kenya´s National Social Security Fund has laid out in its Corporate Strategic Plan 2023-2027 the vision to better serve its current members and to extend social protection coverage, by emphasizing user-centricity and innovation embracing advanced digital technologies. In this context, NSSF aims to review its data landscape to advance to a more data-driven organization. Its administrative data is currently stored in different formats and sources, some of which are not in digital format. This limits the organization’s ability to manage data quality systematically and rigorously. The International Labour Office has supported NSSF in extending social protection to new categories of workers and considering the implementation of new benefit schemes through the projects “Extending Social Protection Coverage to Workers in the Informal Economy in Lao PDR and Kenya and Leveraging Digital Transformation: through South-South Cooperation,” funded by the Government of China and PROSPECTS funded by the Netherlands. These terms of reference refer to the work of a consultant, under these two projects, to analyze and improve the data quality of NSSF, including by applying as immediate remedial measures as possible, and to propose ongoing processes for data quality governance and management to ensure continuous data quality.
Deliverables
Output 1. Data quality assessment report
Data quality assessments establish if the data is fit for its purpose. It is the foundation for improving data quality. The data quality assessment involves creating a stand-alone report that contains evidence of the processes, observations, and recommendations found during data profiling.
Activities
- Review and map data landscape, data catalogues, formats, sources, forms, and means of data access and exchange across the organization, and with external parties.
- Review data and records management policies (data collection, storage systems).
- Integrate data into one data system for analysis.
- Define core information and data needs and compare with the existing data sets for validity and fit for purpose.
- Define a revised master data dictionary (inventory and profile of core business non- transactional data) and key transaction data dictionary.
- Specify a data quality measurement model (dimensions* and metrics, see below)
- Measure data quality against defined data quality dimensions
- Document the results and analyse, especially the issues and pain points, the impacts, and the root causes of problems.
* At a minimum, data quality refers to:
- completeness (avoidance of data gaps),
- conformity (within the set of established standards) or validity,
- uniqueness (no duplicate nor redundant records),
- consistency (data values identical for the same nature of events),
- integrity (correct relationships between data is verified),
- accuracy (compared to authoritative sources),
- correctness (free of errors or mistakes),
- granularity and precision (according to standards)
Data quality can also be assessed according to its reliability, accessibility, traceability, and security.
Output 2. Data quality improvement plan
Activities
- Specify data quality goals and indicators for specific categories of master data
- List and schedule actions to address the root causes of data quality issues. For example, implement data validation rules, correct data errors, standardize data formats, or automate data updates.
- Improvement actions address key dimensions of data quality
- Define and write a data quality improvement plan including.
- Ad hoc immediate corrective measures and preventive controls
- Plans and methods for digitizing paper-based data
- Processes to validate data for standard and format
- Addressing data duplications by specifying unique sources of truths
- Define data catalogs and apply normalization standards to data
- Eliminate unnecessary data by minimizing data retention policies
- Preventing poor-quality and non-validated data from being inserted in the master data system, especially when exchanging data with other organizations
Output 3 Report on the implementation of immediate corrective measures
Activities
- Implement concrete and actionable data cleansing operations to improve data quality
- Write report on the measures implemented
Output 4. Quality data governance and management plan and its implementation report
Activities
- Define and document accountability, roles and responsibilities for data quality management
- Data Management Committees or Groups to manage the quality of the master data.
- Master data management group consisting of data owners and stewards in charge of the data management operations
- Specify data quality business rules to be implemented including routines for measuring and correcting data quality issues
- Improvement actions address key dimensions of data quality
- Define actions over a data cycle: data collection, storage, retrieval, and analysis for management information systems and business Intelligence
- Develop a data quality risk assessment including preventive measures to address acceptable levels of risks
- Define procedures for the management of incidents relating to the quality of the master data
- Support the formation of data quality team, and train/coach senior management and quality data support team on structures, procedures, and routines for managing data quality issues
- Evaluate and propose appropriate industry standard trainings that would help the Fund implement the data strategy both in the short and long term.
Particular attention will be given to the contribution of a digital data driven organization to improving the accuracy, security, timeliness, and openness of access to data at all levels of the organization, building on unique sources of data truth, and on the impact of these changes on increasing the capacity of NSSF governing structures and members to receive up to date information reinforcing the quality of decision making, the transparency and trust in the institution.
Timeline
Month 1
Output 1. Data quality assessment report
Data quality assessment
Month 2 and 3
Output 2. Data quality improvement plan
Data improvement plan
Month 3
Output 3. Immediate corrective measures report
Corrective measures and report
Month 2 and 3
Output 4. Quality data governance and management plan including a data strategy
Data governance and data management plan
Training/coaching
Budget
Output 1. Data quality assessment report: 10 days
Output 2. Data quality improvement plan: 7.5 days
Output 3. Immediate corrective measure: 7.5 days
Output 4. Quality data governance and management plan and its implementation report including a Data Strategy: 10 days
Total: 35 days
Contract duration
The duration of the assignment of this contract is 35 days
Requirements
The consultant should have the following qualifications and experience:
- Professional with at least 10 years of experience in conducting data quality analysis and implementing data quality improvement and data cleansing in social security
- Excellent understanding of Information systems architecture and data quality standards, notably ISO 27001:2022, ISO/IEC 38500, COBIT® 4.1, COBIT® 5 and international practices defined in ISO 8000 and the DAMA-DMBOK2
- Excellent communication and command in written English
How to apply
The deadline for submission of expression of interest is the 27th September 2024. Interested individuals are invited to submit their updated CV and expected rate per day to [email protected] by **17:30hrs (**CEST, Geneva time) on 27 September 2024. Email subject heading should mention “Kenya NSSF Social Security data quality analyst”.